Artificial intelligence (AI) is revolutionizing how companies across various sectors manage and govern their data. As AI becomes more integrated into data-driven processes, updating data governance frameworks to reflect this integration is essential for business success and compliance with regulatory requirements.
Ensuring rigorous standards related to data quality, ethical usage, and privacy protection is now non-negotiable.
#1. The Importance of Data Governance in AI
Given that AI relies heavily on large datasets, establishing comprehensive data governance practices should be a fundamental starting point.
According to Petrus Keyter, a data governance consultant at PBT Group, “AI models need high-quality, well-labelled data to deliver accurate outcomes.” This underscores the necessity for organizations to focus on:
- Data Accuracy: Ensuring data is correct and reliable.
- Data Completeness: Making sure all necessary data is present.
- Data Consistency: Maintaining uniformity across datasets.
Any degradation in these areas can significantly undermine AI performance.
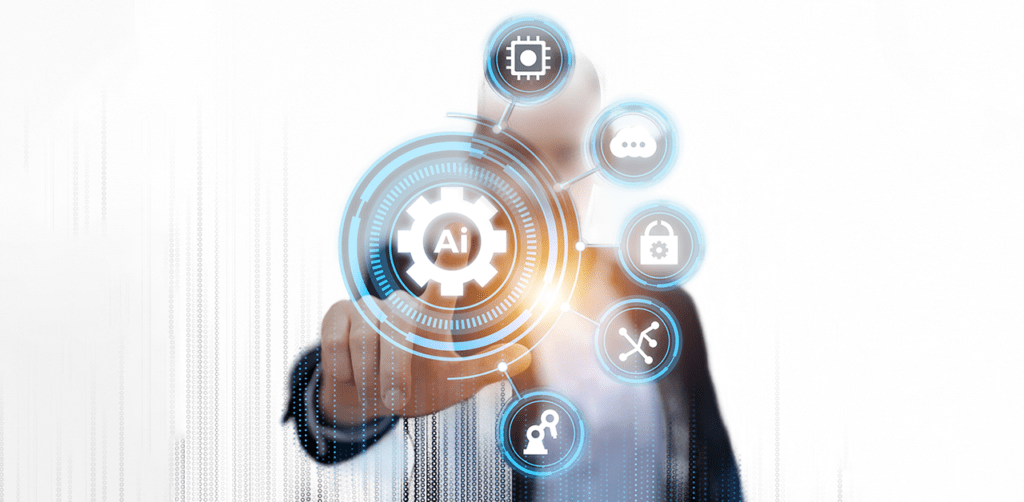
#2. Evolving Data Governance Frameworks
To meet the demands of AI integration, data governance frameworks must evolve. This includes implementing processes for ongoing data validation, quality checks, and error correction. Organizations should prioritize the following components:
- Data Quality Management: High-quality data is crucial for effective AI models. Investing in robust monitoring systems can help ensure that datasets are complete, accurate, and relevant.
- Ethical Guidelines: The ethical challenges posed by AI necessitate guidelines to prevent biases and ensure fairness. Compliance with regulations like the General Data Protection Regulation (GDPR) and the Protection of Personal Information Act (POPIA) is critical.
- Data Security and Privacy: With AI models often handling sensitive information, implementing a detailed security framework is essential. This includes measures like data encryption, access control, and anonymization to protect sensitive information.
- Data Catalog: Within a robust data governance framework, a data catalog serves as a central repository for all organizational data assets. It acts as a single source of truth, providing a comprehensive inventory of data sources, their definitions, quality, lineage, and usage.
- Technology and Architecture: A well-defined data architecture provides a blueprint for how data is collected, stored, processed, and utilized throughout the organization. This includes defining data models, establishing data flows, and selecting appropriate technologies such as data warehouses, data lakes, and cloud platforms.
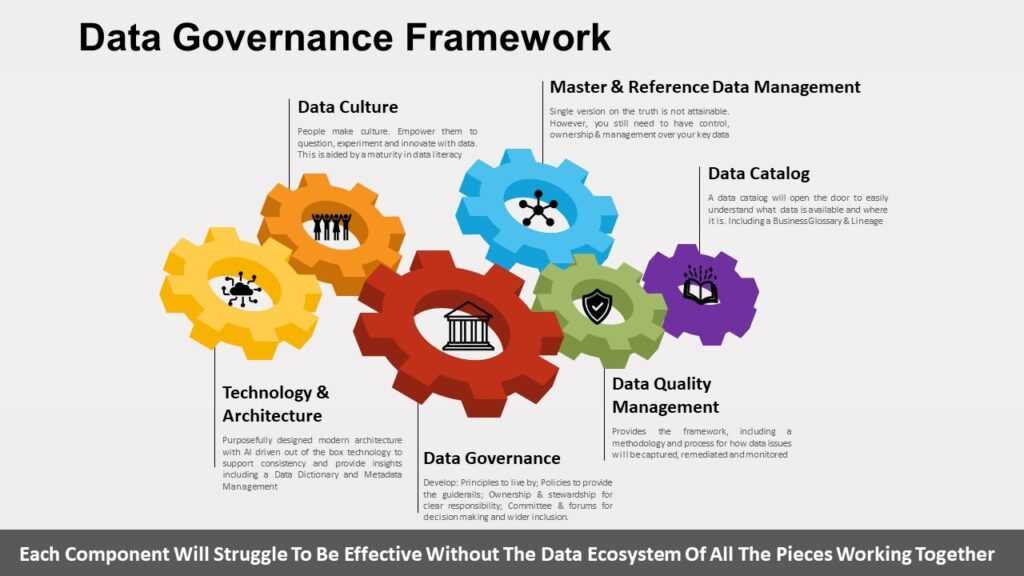
#3. Addressing Biases in AI
AI’s capacity for complex decision-making raises concerns about bias and fairness. Data governance frameworks must incorporate checks for bias to ensure ethical AI usage.
Regular audits and diverse data sampling can help mitigate biases and create more balanced AI models.
Understanding AI Bias
AI bias occurs when an artificial intelligence system produces systematically prejudiced results due to flawed assumptions in the machine learning algorithms. This bias not only affects the fairness of AI-driven decisions but also undermines the accuracy of its outputs.
For instance, a well-known case involved Amazon’s AI recruiting tool, which was scrapped after it favored male candidates based on historical hiring data that predominantly featured men.
Moreover, biases can manifest in critical areas such as criminal justice. The COMPAS algorithm, used to predict recidivism rates, was found to disproportionately flag Black defendants as high-risk while misclassifying white defendants as low-risk.
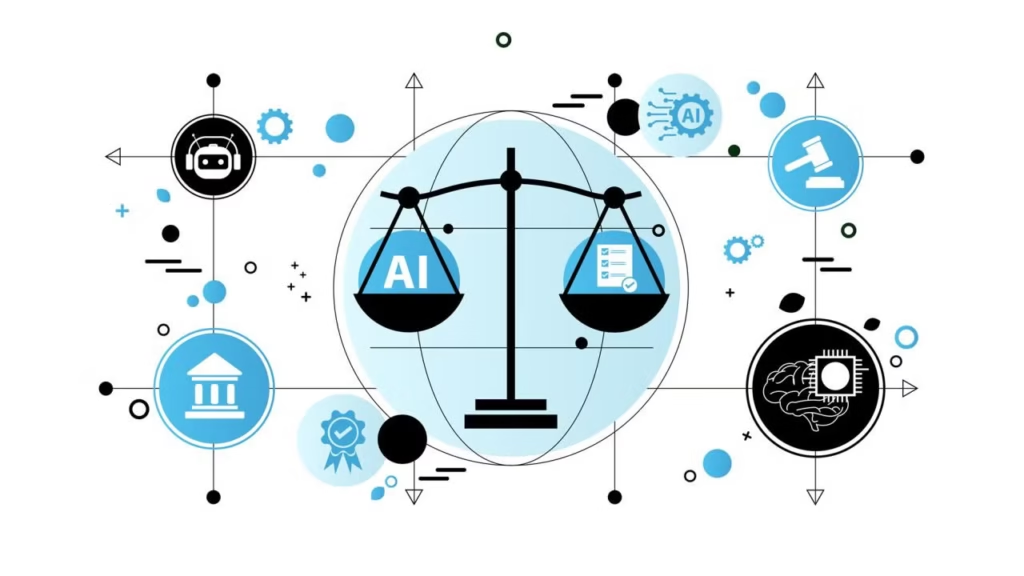
Mitigating Bias Through Data Governance
To address these challenges, organizations must implement comprehensive data governance practices that include:
- Regular Audits: Conducting regular audits of AI systems can help identify and rectify biases. These audits should assess how different demographic groups are affected by AI decisions.
- Diverse Data Sampling: Utilizing diverse datasets during the training phase is crucial. A wide range of scenarios and demographic groups helps ensure that AI models do not favor one group over another.
- Bias Testing: Regular bias testing involves evaluating AI systems against known benchmarks to detect disparities in outcomes across different demographic groups. This process can highlight areas where the AI system might unfairly favor or discriminate against certain groups.
- Algorithmic Fairness Techniques: Implementing algorithmic fairness techniques can significantly reduce AI bias. For example, counterfactual fairness adjusts the algorithm to ensure decisions remain consistent even when sensitive attributes (like race or gender) are altered.
- Human Oversight: While AI can process vast amounts of data quickly, it lacks the nuanced understanding that humans provide. Human reviewers can catch biases that AI might miss and offer context that enhances decision-making quality.
#4. The Importance of Ethical Considerations
Addressing biases in AI is not just a technical issue; it has real-world consequences that can significantly impact individuals and society. Biased AI systems can perpetuate existing inequalities and unfairly affect marginalized communities. This not only erodes public trust but also poses legal risks for organizations using biased systems.
Incorporating ethical guidelines into data governance frameworks helps prevent unintended biases and ensuring compliance with regulations like GDPR and POPIA. Regular audits and stringent data usage protocols can help companies align their AI practices with legal standards and customer expectations.
#5. The Role of Transparency in Data Governance
For AI systems to operate transparently and accountably, companies must track the flow of data from its source to its transformation and eventual use in AI applications.
This transparency is crucial for regulatory compliance and troubleshooting. Utilizing data lineage tools allows organizations to trace issues back to their source, enhancing the reliability of AI-driven outcomes.
Privacy-by-Design Principles
Given the sensitive nature of the data used in AI applications, privacy protocols must be stringent. Implementing privacy-by-design principles ensures that role-based access controls, anonymization techniques, and encryption are in place to safeguard data integrity source.
Conclusion
Building a comprehensive data governance framework that considers these factors is key to unlocking the full potential of AI while mitigating risks related to data quality, ethics, and security.
As AI continues to evolve, so too must the frameworks that govern it. By integrating these principles into their operations, businesses can leverage AI responsibly, creating impactful solutions that drive meaningful insights and decision-making.
Read More: